The AI in Finance Summit brought together both senior leaders and AI practitioners from the Finance sector. More than 20 speakers covered topics including AI use cases in Finance, the power and risks of Generative AI (GenAI), and the need for building in human supervision in AI systems. Below is a summary of what I heard and my own take on the future of AI in Finance.
Balancing speed, data privacy, and accuracy
A senior leader Raymond James reminded the audience about the importance of striking a balance between speed and accuracy when it comes to innovation. They presented the AI-assisted automation formula as the combination of timely information retrieval with augmented human decisioning, both leading to faster and better decisions. They walked audiences through the evolution of AI in the Finance sector and cautioned about the pitfalls such as the importance of data safety, security and privacy. In this subject, innovators at TD said they were looking at implementing Large Language Models (LLMs) on-prem in a secure way, eliminating the need to send data to external parties.
Real world examples
JPMorgan Chase showed a presentation titled “The Evolution of Artificial Intelligence in the Finance Industry”. The presentation included a timeline showing the introduction of AI in the finance sector starting in 1980s; an overview of use cases in banking, corporate finance, trading, fintech and insurance; real world examples; key statistics; and future trends. The real word examples covered implementations by some of the leading financial organizations, including:
- JPMorgan Chase implemented a contract intelligence tool that automates the review of legal documents such as loan agreements and derivatives contracts, extracts key datapoints, and reduces the time and effort required by lawyers and compliance professionals.
- Robinhood uses AI to analyze market data, identify opportunities, and enable users to execute trades quickly and easily.
- BlackRock uses AI to analyze market trends, portfolio performance and risk factors to help investors make informed investment decisions and manage risk.
- PayPal has more than 400 million accounts, therefore it must implement robust fraud detection measures to protect users. The company uses AI to analyze transactions in real time, identify suspicious activities and patterns, and flag potentially fraudulent transactions.
- HSBC launched an AI-powered chatbot to assist customers with inquiries related to account balances, transaction history, and account management. The tool is aimed at enhancing the customer experience and reducing the need for human intervention in customer service.
This specific presentation made no mention of GenAI at all (this was refreshing). When asked about GenAI, the presenter said that “GenAI is still a few years away from being launched broadly in production”.
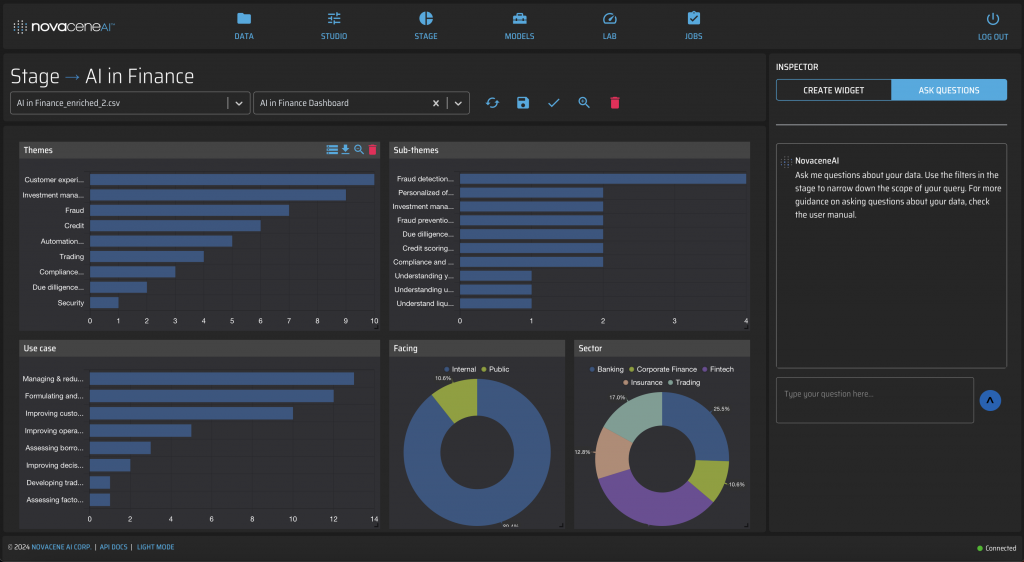
Figure 1: The NovaceneAI Platform reveals the dominant topics from the conference. Customer experience is number one.
Enhancing the customer experience
Enhancing the customer experience was the most talked-about use case at the summit.
The Vice President of AI & ML Research at one of the largest Financial Services companies showed practical examples of how GenAI can be used for content personalization. They explained how a 401K can be explained to younger audiences using a sports metaphor. They also talked about how GenAI can be a brainstorming partner to help users come up with compelling content when writing reports for the executive team.
None of these uses of GenAI should be carried out without care, they cautioned—it is essential that those of us using GenAI at work are able to verify and validate the content that LLMs generate. LLMs are known to hallucinate—the name given to the technology’s vulnerability to confidently assert inaccurate information. Without proper guardrails provided by an effective Human In The Loop feature, it will be too risky to rely on these artificial co-pilots for business critical decision-making.
They also covered other interesting use cases, such as the way LLMs could revolutionize user experience (UX) and user interface (UI) techniques that web designers use to help users find content: they reminded us how Google revolutionized search by simplifying the experience down to a single text field. Or how robust search systems could facilitate finding experts across the organization with whom to collaborate.
Lastly, they explained the advantages of looking at other industries such as healthcare or education for sources of inspiration. For example, in healthcare, there are parallels between the patient-doctor and customer-financial advisor relationship that can help organizations devise novel ways to improve the experience of their customers.
They concluded acknowledging the need to for organizations to innovate quickly: “Customers already have access to GenAI, so financial institutions must get ahead of the game” they said.
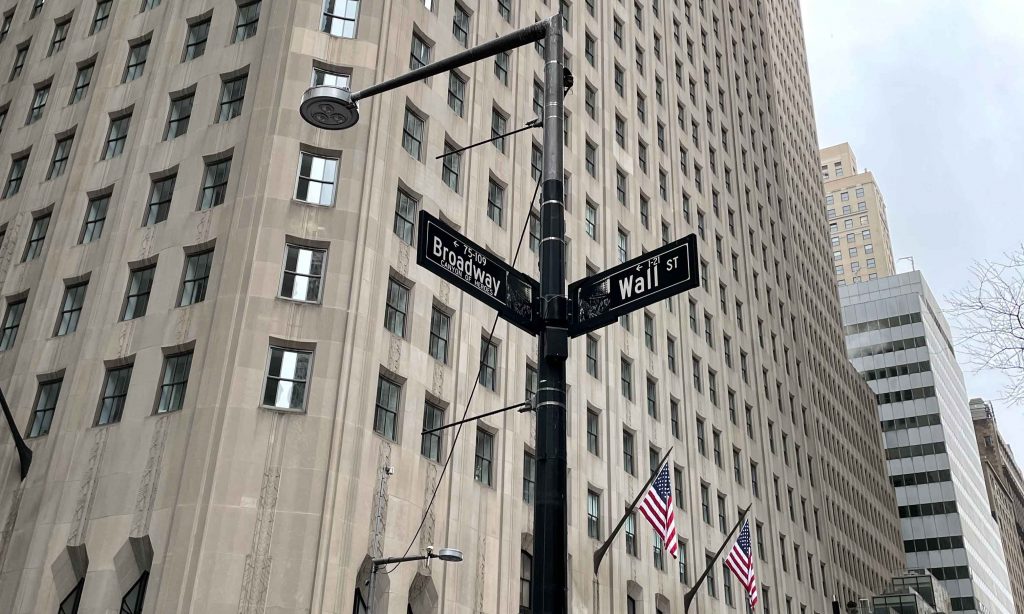
Knowing the customer
A clever use of GenAI was solving data scarcity when it came to understanding people’s spending patterns. Although Mastercard processes a staggering 140 billion transactions per year, this number only represents transactions that were done using their products. This creates a data scarcity problem, where analysts only have a partial picture. Using a clever example, the Director of AI Products at Mastercard demonstrated how humans are able to figure out known objects—such as a dog—from incomplete images. They explained how GenAI could be used to “fill in the blanks” and solve data scarcity problems in the real world.
Process automation and efficiency
During a breakfast break, I met the Global RFP Manager at Mackenzie Investments. They lead proposal writing at Mackenzie, coordinating the writing of proposals to respond to RFPs. He was interested in hearing about solutions that would help them automate the writing of these proposals.
How GenAI and NLP support automation
There is no shortage of applications of natural language processing (NLP) in Finance. From customer service chatbots, to analyzing the text in the descriptions of transactions, NLP enables organizations to understand their customers, keep them safe, and improve the quality of the services they receive.
An AI & ML researcher from Acorns, delivered a very practical presentation on using LLMs to perform traditional NLP tasks, including sentiment analysis, named entity recognition, and topic classification. This is a trend we are seeing at NovaceneAI as well, where we are carrying out the same kind of experiments. We are seeing that GenAI is remarkably capable of delivering impressive results, surpassing the quality and nuance of their NLP counterparts.
A Vice President at JPMorgan Chase, which holds 80 million accounts globally, demonstrated practical uses of text summarization with T5 transformers—a pre-ChatGPT technology. The presentation highlighted the effectiveness of fine-tuning in enhancing the performance of generic models for specific applications like dialogue summarization. Additionally, they emphasized the importance of defining use case scopes and experimenting with various techniques such as prompt engineering and zero- or few-shot learning before committing to extensive and costly fine-tuning.
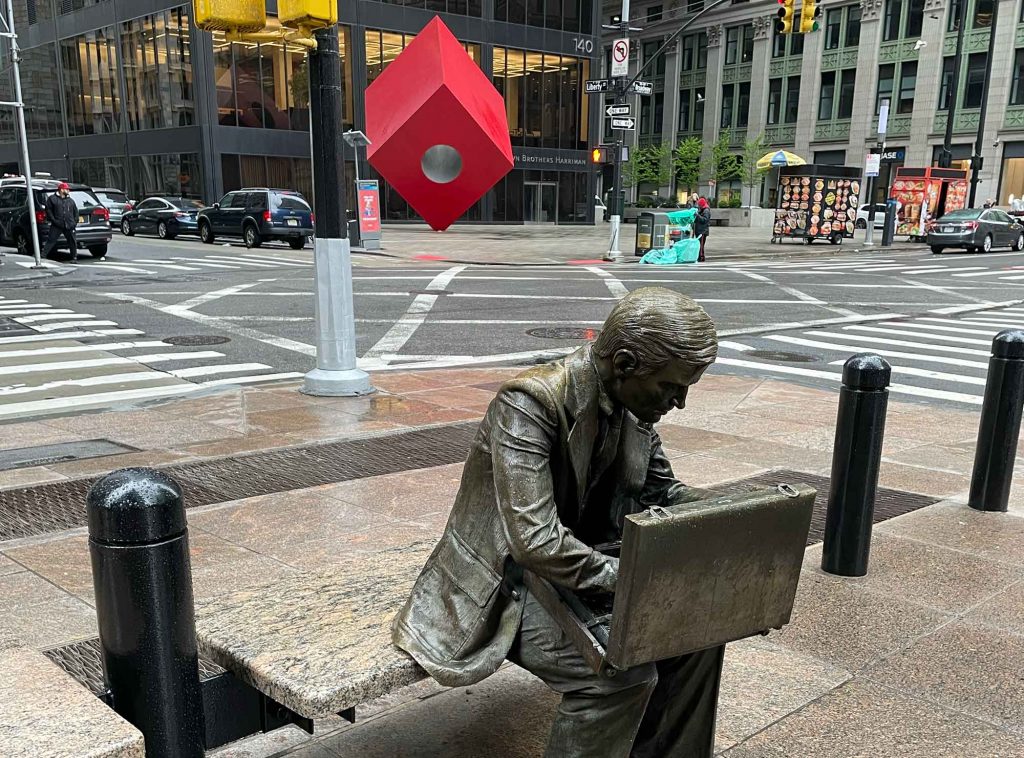
The future is customer-facing
In the past, banks used AI for loan and investment recommendations. Now, they are more focused on the customer because all other tasks have largely been automated.
When speaking about future trends, JPMorgan Chase mentioned the following use cases:
- Decentralized Finance (DeFi)
- Hyper-personalized financial services
- AI-powered quantum computing
- Explainable AI
- AI-driven financial wellness and education
- AI-augmented decision-making for traders and investors; and
- AI-powered autonomous financial agents
A Director of AI Products at one of the largest credit card companies said that built-in explainability, and allowing a wider range of people in the organization to use AI through user-friendly AI tools were two of the most important future trends.
Conclusion
In closing, the key, most talked about topics included human-in-the-loop, explainability, the need for user-friendly AI tools, and the importance of reducing hallucination. Human-in-the-loop systems must put the subject matter expert back in the driver’s seat: these experts are the ones who best understand the nuance of their practice, and therefore having experts define the business questions that AI must help answer will increase the likelihood of success. The combination of these factors will enable organizations to strike the right balance with between speed, privacy and accuracy of their AI-driven innovations, deliver measurable ROI, and enable productive collaboration between the business and IT.